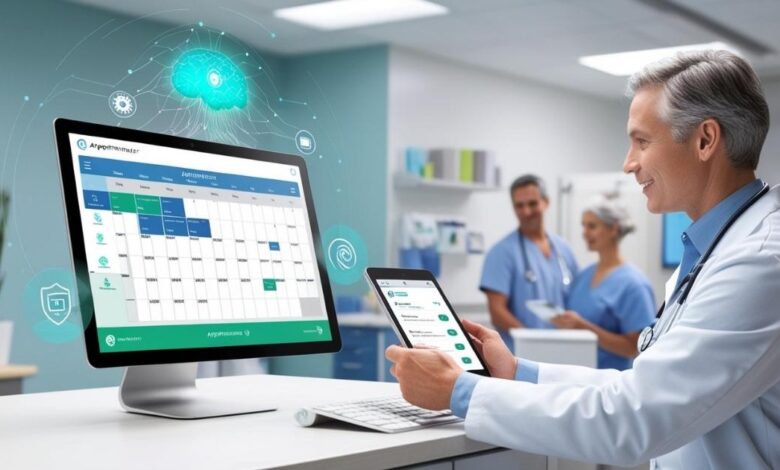
How AI predicts and manages appointment cancellations
How AI predicts and manages appointment cancellations, Time no-shows and cancellations of appointments are some of the complex problems that affect providers of healthcare services all over the world. These disruptions not only cost-minimizing but also burden the health system and they also have an impact on health care delivery to patients.
An example of such events has been the challenge that providers have faced when trying to forecast and control these cancellation situations, and this for years, mostly, relying on simple call and reminder systems. But unlike earlier approaches, with AI, a much richer solution is available today.
It has become easier now that artificial intelligence helps in creating predictive models to identify cases of no-shows or cancellations before they occur thus helping healthcare providers work better at arranging their timetable adequately.
Key Takeaways
- In addition, through AI-powered systems, it is possible to predict and work with the cancellation of appointments and avoid no-shows.
- Using hopes from previous years, the AI models are useful when it comes to scheduling by the health care providers.
- Presenting real-time information and avoiding last-minute cancellations, the application is based on predictive models using machine learning. ‘
- AI is beneficial in patient care because it gives better predictions about the next appointment and cuts out waiting time.
- AI solutions can also be easily interfaced with the current scheduling systems, to improve appointment management.
Understanding the Problem: No-Shows and Cancellations in Healthcare
The Impact of No-Shows on Healthcare Providers
Studies have shown that missed appointments are not a rarity in the sphere of healthcare and they can potentially be dangerous for everybody involved.
This is called gap concerns in care if a patient fails to attend an appointment with a provider without any cancellation. This interrupts not only the healthcare provider’s working time but also the process of patient treatment.
Consequences of No-Shows
- Wasted Resources: This paper will also address how, when a patient misses an appointment with a healthcare provider, the health facility or provider loses time, human resources, and diagnostic tools that had been reserved for that particular appointment.
- Impact on Patient Care: According to the findings, a no-show of patients could result to a longer cycle of treatment for other clients hence a low availability of the clinics.
- Revenue Loss: To healthcare providers, arrive lest you want to lose your hard-earned money on no-shows. Appointments cannot be made on short notice when they have been scheduled and missed.
- Increased Pressure on Scheduling Systems: He stressed that healthcare providers may experience a lot of anxiety when organizing their schedules in order to fill blanks in their calendars.
Traditional Methods for Managing Cancellations
Originally, the task of managing missed appointments came with a system of using manual tracking methods. This meant relying only in phone calls, emails, or written and pasted schedules to manage the appointments and cancellations.
Challenges of Traditional Scheduling
- High Administrative Workload: Organization of staff involving sending reminders to patients that appointments have been rescheduled or making follow-ups for appointments was done through a process that would require everybody to do it on their own and this was very tiresome besides being uncharted for most of the occasions.
- High Missed Appointment Rates: Even with various reminders and follow-ups made there were still many a healthcare provider who missed many appointments.
- Limited Prediction Ability: There was no way of knowing days, hours or even minutes in advance that a given appointment would be canceled or a patient would fail to show up.
How AI Predicts and Manages Appointment Cancellations
The Role of AI in Predicting No-Shows
The use of artificial intelligence and machine learning presents the best solution to the situation whereby patients fail to attend their appointments. Using statistical data AI models are able to determine who of the patients is likely to not attend the appointment.
Predictive Models in Healthcare
It is through the application of predictive analytics that AI can be able to work out the behavior of patients. It looks at various factors such as:
- Past appointment history
- Frequency of cancellations
- Patient-related factors (age, gender, location)
- Time of the appointment
Predicting Cancellations with AI-Driven Algorithms
AI does not have a simple stab in the dark when it comes to guessing which persons might miss an appointment; instead, it predicts. Such predictions make it possible for healthcare providers to act proactively such as sending a gentle reminder or suggesting other convenient times.
How AI Can Predict Patient No-Shows
By analyzing data such as:
- Personal details and medical history (whether the patient has been neglecting appointments in the past?)
- Booking behavior indicates how far in advance an appointment was booked or how much advance notice of cancellation is given.
- Reminder response (did the patient get a previous reminder and respond?)
It can predict whether a patient is capable of showing up to a session or whether the patient is going to bail at the last minute. This makes the prediction model for the amount of rewards to offer better with time as the AI is trained on fresh data.
Integrating AI Into Scheduling Systems
Actually, the implementation of AI in scheduling algorithms doesn’t disrupt the functioning of healthcare software. A predictive system for cancelations is integrated into them, so they can adjust schedules in real-time, perchance the cancelation of an appointment, without wasting the healthcare provider’s time.
AI-Powered Scheduling Tools
- Automated Appointment Reminders: AI systems use email or text alerts, or even make phone calls in order to ensure that the appointment is not forgotten.
- Rescheduling Made Easy: When there is a cancellation, then the AI system advises the patient on other suitable dates thus minimizing space in the calendar.
Machine Learning: A Deep Dive into Prediction Models
How AI Models Are Built to Predict No-Shows
Machine learning is the key component of AI models intended for the prediction of no-show patients. These models are optimized for the purposes of data analysis and making sense of massive datasets to make predictions about them.
Using Historical Data for Predictions
Machine learning models learn from past behavior, like:
- Whether a patient had his or her last appointment canceled.
- Depending on whether the patient has a history of late cancellations.
- In what time prior did, the specific appointment was made?
Types of AI Models Used in Healthcare
Supervised Learning
- AI is trained in a set of examples in which some of the. appointments are canceled, others are not. In this way, I can predict future outcomes based on the outcome of data that the AI learns.
Unsupervised Learning
- Other AI models may also try to search data dependencies in a set of data that was never tagged for cancellation, which would pinpoint potential cyclical patterns that can be used for prediction.
Hybrid Approaches
- Supplementing the table learning with the machine learning – this let the AI system become more precise in the no-show prognosis, especially when applied to a complicated case.
Predicting Last-Minute Cancellations
AI models can also predict the chances of cancellation as a problem that is very hard to solve for the providers. There is an ability to analyze patterns to determine when a patient will likely cancel soon before the appointment so that the necessary steps can be taken by the providers.
Benefits of AI in Appointment Management
Improving Efficiency in Appointment Scheduling
AI not only can predict no-shows but can also aid in painting the most efficient picture of the schedule. It follows that the analysis of appointment data allows AI to recommend the best time slots for patients to be taken, hence increasing appointment productivity.
Optimizing Appointment Slots
AI is used to forecast the most opportune timings of the appointments depending on such routines that existed prior. This feature can help decide when a given patient is most likely going to arrive and also prevent cases where there are gaps in busy surgery schedules.
Enhancing Patient Care with AI
It is not only beneficial for the providers to predict no-shows and to automate appointment management but it is also helpful to patients. The use of artificial intelligence also means that schedules are fully booked and patients get prescribed care quickly.
Better Access to Healthcare
Even when patients ‘cancel their appointments, AI is able to forecast this thus ensuring that filled slots are reduced note of the time patients spend waiting for care. This nets out on improving access to various types of healthcare services.
Reducing No-Show Rates with AI
By promoting timely appointments, constant notifications, and working in advance for canceling sessions, no-shows can be minimized through AI.
Automated Reminders and Alerts
- Text and email reminders
- Voice AI systems for subsequent calls in the form of reminder call
This has a positive implication for the improvement of health care since this minimizes the chances of no-shows among patients.
AI Tools and Technologies Used for Appointment Prediction
Exploring AI Technologies in Healthcare
This shows that not only does AI have a role in more than just reminding patients of appointments. It applies sophisticated algorithms and probabilistic decision-making models to predict the future behaviors of patients on the system quite accurately.
Artificial Intelligence and Machine Learning
AI is intended for data handling, analysis of big amounts of data in terms of trends, and making the correct prognosis humans can hardly do.
AI Solutions for Appointment Scheduling
Today, many providers are using an AI-powered scheduling system that easily interfaces with other programs they have. These systems use various aspects of the patient’s information to determine which time slots would be most suitable for appointment hence maximizing the schedule’s blanks.
AI-Powered Scheduling Systems
- It is used to estimate the availability and also optimize the appointment as well.
- Cuts on administrative burden
- Saves time that could be used in following up on patients.
AI for Personalized Healthcare Management
AI does not only predict population trends but it also predicts trends for an individual patient. AI can use appointment history sorts their preferences, and provide a perfect appointment timetable that would be perfect for attendance.
The Future of AI in Healthcare Appointment Management
The Evolving Role of AI in Healthcare Systems
AI is changing the face of the healthcare system, and more practices are implementing AI for the management of appointments daily. AI models will also help to define when customers are likely to cancel or fail to attend the meeting, thus also improving given that models learn from an increasing amount of data.
AI-Powered Evolution
Healthcare appointment scheduling of the future will involve the use of intelligent systems that improve with age. With these systems becoming more advanced, future predictions are sure to get even closer to the real thing, thus greatly reducing missed appointments.
Integrating AI with Conversational AI
AI is also entering in the conversation systems. The uses of Voice AI systems allow them to communicate with patients to book an appointment or remind them via a call or even a message.
AI Agents for Personalized Interactions
- AI agents will be able to handle complex interactions for the patients and patients will be able to get a personalized experience.
- Apart from text, patients are also offered voice AI in case they do not like the text kind of reminders.
Case Studies: Successful Implementation of AI in Healthcare Appointment Management
Real-World Examples of AI Reducing No-Shows
Case Study 1: AI in a Large Healthcare Network
An extensive healthcare network adopted an AI-based digital solution for two key services; appointment booking demonstrated a no-show decrease of over 30%. The system managed to fill some missing appointments in real time because of anticipating no-shows through suggestions of targeted reminders.
Case Study 2: AI in Small Healthcare Practices
A clinic with fewer patients applied an AI model to predict no-show patients by and large. It also helped in the proper administration of clinical appointments and enhanced the access of patients and a number of persons treated.
FAQs about How AI predicts and manages appointment cancellations
Q1. How does AI predict appointment no-shows?
AI uses historical data, such as past appointment history and patient behavior, to make predictions about future cancellations.
Q2. Can AI automate appointment reminders?
Yes, AI can send automated reminders via email, text, or voice notifications to reduce missed appointments.
Q3. Is AI really effective in reducing no-show rates?
Yes, AI has been shown to reduce no-show rates by accurately predicting cancellations and sending timely reminders to patients.
My Opinion
In my view, focusing on the company of canceled appointments is one of the greatest opportunities of AI in the sphere of medicine. The fact that patients’ behavior can be forecasted with the help of a predictive model will also be profitable because time and resources have been spent on patients’ no-shows before.
Machine learning makes it possible for the entire scheduling for such affairs as appointments to be conducted either through notification or changing schedules in the shortest time possible with little influence from human beings.
Personally, I do not think that AI integration into appointment systems will only provide more efficiency, but also a better experience for the patient. The experiences of patient care will be less interrupted by attendance problems, patients will be seen faster, and appointment scheduling will be more dependable as a result.
With time, I believe that there will be enhanced individual means of arranging for appointments hence an enhanced and moreolithic patient convenient healthcare.